Data Feeder – Warehouse
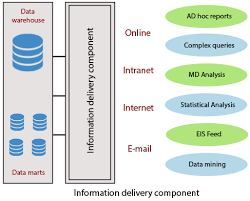
In the digital age, data reigns supreme. Every decision, every innovation, and every strategy is backed by a wealth of information. Consequently, the need for robust data management systems has become paramount. Among the various components of this data ecosystem, two pillars stand out: Data Feeders and Data Warehouses. Together, they form the backbone of efficient data utilization and analysis for organizations across industries.
Understanding Data Feeders and Data Warehouses
Data Feeders:
Data Feeders, often referred to as data pipelines or ingestion systems, are the mechanisms responsible for collecting, processing, and delivering data from diverse sources into a centralized location. These sources can range from databases, APIs, streaming services, IoT devices, social media platforms, and more. The primary objective of data feeders is to ensure that raw data is cleansed, transformed, and standardized before being loaded into the data warehouse.
Data Feeders serve as the bridge between the myriad of data sources and the analytical capabilities of the organization. They perform tasks such as data extraction, data transformation (ETL – Extract, Transform, Load), data validation, and data enrichment. Additionally, they often incorporate features like data quality checks, error handling, and scheduling to ensure a seamless flow of information.
Data Warehouses:
On the other hand, Data Warehouses are specialized databases designed for storing and analyzing structured and unstructured data. Unlike traditional transactional databases optimized for quick data retrieval, data warehouses prioritize query and analysis performance. They utilize various optimization techniques such as indexing, partitioning, and clustering to expedite analytical queries over vast datasets.
Data Warehouses typically follow a schema-on-write approach, meaning data is structured and organized before being loaded into the warehouse. This organized structure facilitates efficient querying and analysis, enabling businesses to derive valuable insights and make informed decisions. Common features of data warehouses include data compression, columnar storage, and support for complex analytical functions.
The Integration: Data Feeder – Warehouse
Seamless Data Flow:
Integrating Data Feeders with Data Warehouses creates a seamless data flow pipeline within an organization. Data Feeders ensure that raw data from disparate sources is efficiently processed and transformed into a consistent format compatible with the warehouse schema. This standardized data is then loaded into the warehouse, where it becomes readily available for analysis and reporting.
Real-time Updates:
In many modern applications, real-time data processing is crucial for timely decision-making. By integrating Data Feeders with Data Warehouses, organizations can achieve near real-time updates of their analytical data. Streaming data from sources such as IoT devices, social media feeds, or transactional systems can be ingested, processed, and immediately made available for analysis, enabling businesses to react promptly to changing conditions.
Scalability and Performance:
Scalability is a key consideration for any data management system. By integrating Data Feeders with Data Warehouses, organizations can scale their infrastructure according to the growing volume and complexity of data. Modern data warehouses offer horizontal scalability, allowing organizations to seamlessly add more compute and storage resources as needed. This ensures that analytical workloads can be processed efficiently, even as data volumes continue to increase.
Data Quality and Governance:
Data quality and governance are paramount in ensuring the reliability and integrity of analytical insights. Data Feeders play a crucial role in enforcing data quality standards by performing validation checks, cleansing processes, and error handling before data is loaded into the warehouse. Additionally, by centralizing data ingestion through Data Feeders, organizations can implement consistent data governance policies, ensuring compliance with regulatory requirements and internal standards.
Cost Optimization:
Integrating Data Feeders with Data Warehouses can also lead to cost optimization. By streamlining the data flow pipeline and reducing the need for manual intervention, organizations can minimize operational overheads associated with data ingestion and processing. Furthermore, modern cloud-based data warehouses offer flexible pricing models, allowing organizations to pay only for the resources they consume, thus optimizing costs based on actual usage patterns.
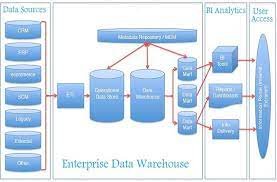
Case Study: Retail Analytics
Consider a retail organization that operates both online and brick-and-mortar stores. To gain insights into customer behavior, sales trends, and inventory management, the organization integrates its various data sources, including point-of-sale systems, e-commerce platforms, social media channels, and supply chain databases.
Data Feeders are deployed to extract data from these diverse sources, perform necessary transformations and validations, and load the cleansed data into the organization’s Data Warehouse. Real-time data streams from online transactions and social media interactions are processed alongside batch data from store sales and inventory databases, ensuring a comprehensive view of the business operations.
Once the data is loaded into the Data Warehouse, retail analysts and business stakeholders can run complex queries and generate reports to identify key performance indicators, forecast demand, optimize inventory levels, and personalize marketing campaigns. By leveraging the integrated Data Feeder – Warehouse solution, the retail organization can make data-driven decisions swiftly, enhancing operational efficiency and driving revenue growth.
Conclusion
The integration of Data Feeders with Data Warehouses represents a symbiotic relationship that underpins modern data management practices. By seamlessly connecting data sources with analytical capabilities, organizations can unlock the full potential of their data assets. From real-time insights to scalable infrastructure and cost optimization, the benefits of this integration are manifold. As businesses continue to navigate the complexities of the digital landscape, the synergy between Data Feeders and Data Warehouses will remain indispensable in driving innovation, agility, and competitive advantage.